PointResNet - Residual Network for 3D Point Cloud Segmentation and Classification
- Designed a residual-block based novel architecture that outperformed the baselines by 4% for the segmentation task on ShapeNetPart dataset and produced comparable results for the classification task on the ModelNet-40 dataset.
- The network takes n input points and applies transformations, several multi-layer perceptron (MLP) layers with skip connections, and then aggregates features using max pooling. The classification network applies fully connected and dropout layers and obtains k scores for k classes. The segmentation network extends the classification network by combining local and global features, using convolutional layers, and finally giving per point scores as output. The connected skip layers represent ResBlock-n, where n is the number of features of a single block layer. Numbers in MLP layers represent a number of features.
Quick links:
Architecture
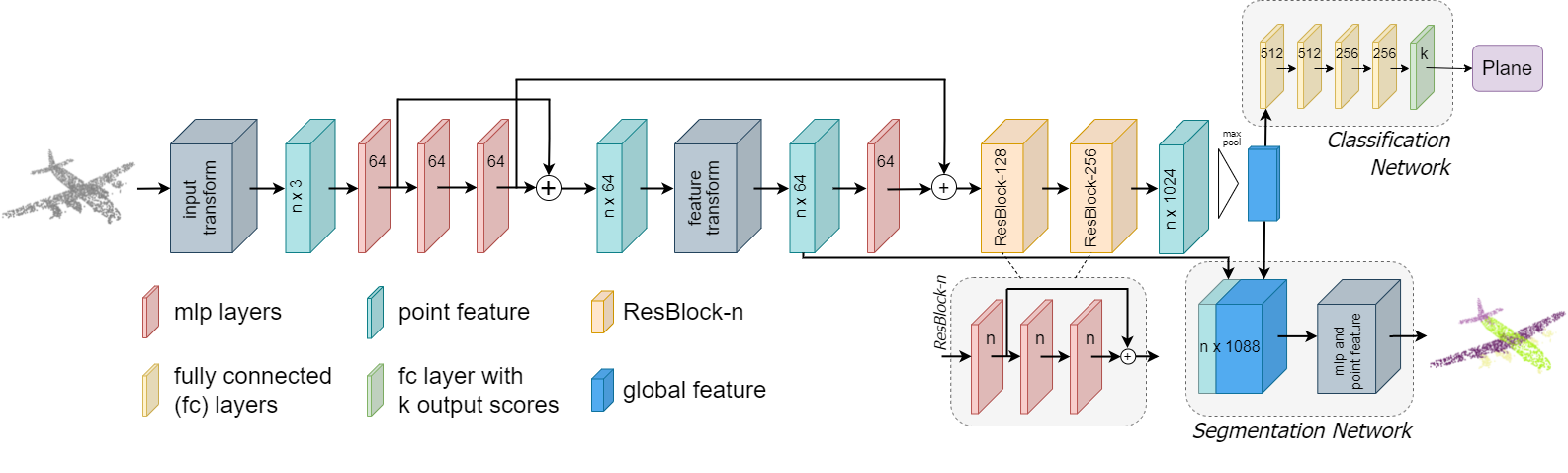
PointResNet architecture
Results
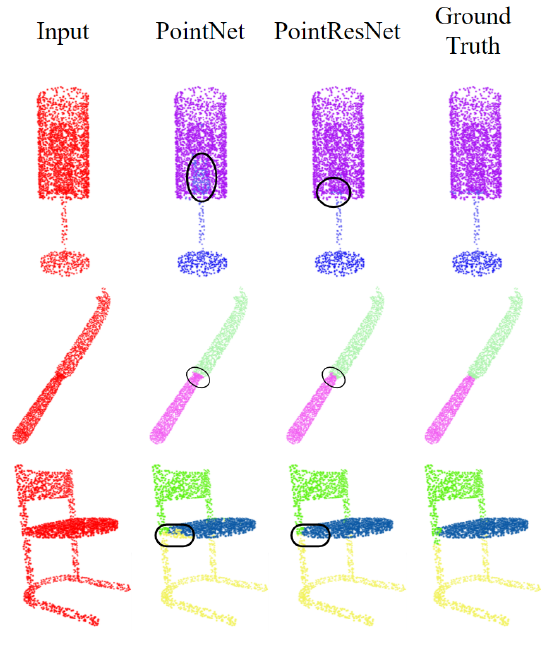
Qualitative comparison of part segmentation results on ShapeNetPart dataset
Eval acc ↑ | |
PointNet | 90.6 |
PointNet++ | 88.4 |
ECC | 90.8 |
RTN | 92.6 |
PointResNet (Ours) | 94.79 |
Quantitative comparison of part segmentation results on ShapeNetPart dataset
ModelNet10 | ModelNet40 | |||
---|---|---|---|---|
Eval acc | Eval avg class acc | Eval acc | Eval avg class acc | |
PointNet | 92.52 | 92.08 | 89.2 | 86.2 |
PointNet++ | - | - | 91.9 | - |
PointConv | - | - | 92.5 | - |
ECC | 90.0 | - | 83.2 | - |
RTN | - | - | 90.2 | 86.5 |
ResNet-50 | - | - | 66.3 | - |
PointResNet (Ours) | 92.86 | 92.29 | 88.76 | 85.58 |